OpenDoor: applying big data to home selling
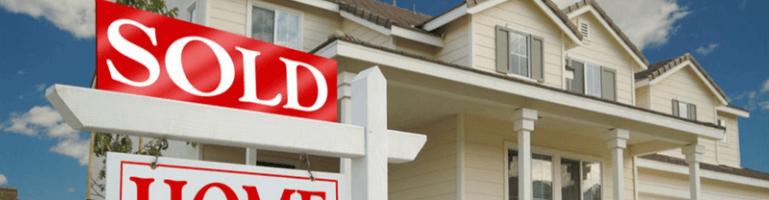
Using the power of data analytics, OpenDoor will offer you a price for your house in under 24 hours
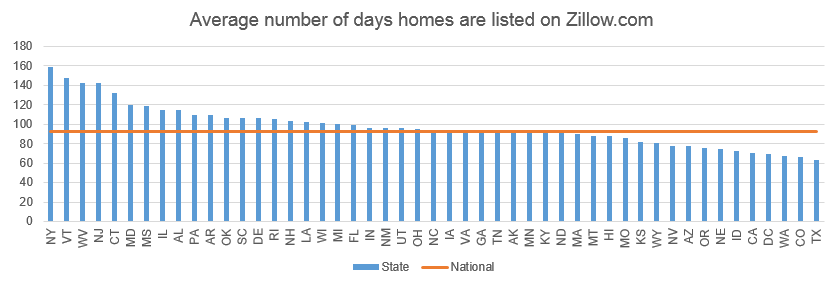

Selling a house can be a source of deep frustration. According to real estate website, Zillow.com, the average US home spends three months listed on the market (see ex. 1).[1] A large cause of the delay is the difficulty of information discovery. Home buyers need to physically visit the properties, assess the various features, and somehow conjure up a price. It’s a huge challenge for any individual buyer. But what if you could utilise the data from thousands of previous transactions? That’s where OpenDoor comes in.
OpenDoor uses the power of big data to calculate home values, fast. They offer to buy properties from sellers quickly in exchange for a fee. OpenDoor then resells the houses, keeping the fee and a small margin.
Value Creation and Capture
Real estate investors and lenders have been using automated valuation
models (AVMs) to model house prices for quite some time. These AVMs usually consist of two parts: a repeat-sales index (which estimates house prices changes over time) and a hedonic pricing model (which estimates a property’s value by piecing together its constituents – bedrooms, bathrooms, etc.). The literature on both methods is extensive.[2] Nonetheless, building an accurate AVM is still a real challenge. For example, in Zillow’s infamous Zestimate, 55% of valuations are more than 5% off from the actual sale price (see ex. 2).[3] CoreLogic, a leading provider of AVMs, is more than 15% off from the sale price in 10%+ of cases.[4]
To enhance the accuracy of its AVM, OpenDoor’s team of data scientists have had to do things differently:[5]
- Getting super specific on inputs – in addition to considering standard house features, OpenDoor’s model also uses very granular (often proprietary) inputs such as the property’s proximity to freeways, curb appeal, and kitchen countertop material[6]
- Narrowing focus to where the model is most reliable – OpenDoor only works with single-family houses built after 1960, priced between $125,000-500,000 in cities where housing is more homogenous (e.g., Phoenix, Dallas)[7]
- Understanding second-order relationships – rather than just consider the direct impact of inputs on price, OpenDoor attempts to understand how inputs interact with each other, for example, a pool adds more or less value depending on the quality of the neighbourhood
The resulting reliability gives OpenDoor the confidence to make offers to sellers in as little as 24 hours.[8] Providing sellers this speed and convenience then allows OpenDoor to capture value in three ways:
- Charging a 6% service fee akin to a real estate agent
- Pricing slightly below market value (OpenDoor estimates their offers are 1-3% below market,[9] others estimate 6%[10])
- Adding 0-6% in fees for resale risk, based on market conditions[11][12]
Unlike other bulk house buyers such as We Buy Ugly Houses, OpenDoor doesn’t focus on major renovations and ‘flipping’ the properties. Instead, their model is one of scale – buy and sell, earning a small sliver on each transaction.

Challenges and Opportunities
Few others have dared to utilise such a purely data-driven approach in real estate. Getting the model right is an enormous challenge. Accessing accurate and granular data at scale is difficult (many inputs required physical validation), and even small errors on such a large capital base can be devastating. Moreover, the risks associated with wide-scale systemic downturns is nearly impossible to factor in.[13] As it expands, OpenDoor will face additional challenges such as whether it can offload the properties acquired quickly enough and whether its model can be adjusted for new markets.
However, the potential upside from cracking this nut is immense: real estate broker revenues top $100bn a year in the US alone.[14] OpenDoor also has the opportunity to take a slice of other components of the real estate transaction: generating leads for preferred lenders; operating SaaS for appraisers; sharing information with real estate databases.
If OpenDoor is successful, maybe one day we’ll see an efficient market for real estate emerge, and selling a house will be as easy as clicking a button.
[1] https://www.zillow.com/research/data/ Days on Zillow dataset; average of 2016
[2] One Texas study calculated that golf courses increase nearby property values by 26% http://journals.humankinetics.com/doi/pdf/10.1123/jsm.21.4.555
[3] http://www.realestatedecoded.com/zillows-typical-error/
[4] https://www.corelogic.com/imgs/international/retrospective-avms.pdf
[5] https://www.forbes.com/sites/amyfeldman/2016/11/30/home-shopping-networkers-opendoor-is-upending-the-way-americans-buy-and-sell-homes/#1dc273c3430c
[6] OpenDoor asks sellers to provide specific inputs not available in existing databases. A physical inspection is also conducted, though the offer price is typically made before this inspection.
[7] Interestingly, the three cities where OpenDoor currently operates, Phoenix, Dallas, and Las Vegas, are in states where the average listed time is below the US average. This lower listing time may reflect the more ‘standardised’ nature of housing there
[8] https://www.opendoor.com/faq/seller/the-opendoor-offer-seller
[9] https://techcrunch.com/2016/06/07/a-startup-that-pays-cash-to-buy-homes-now-offers-money-back-guarantee/
[10] https://www.inman.com/2017/03/22/opendoor-cost-markup-fee-las-vegas/
[11] https://stratechery.com/2016/opendoor-a-startup-worth-emulating/
[12] https://www.opendoor.com/pricing
[13] Some speculate that OpenDoor will increase its ‘risk fee’ in a market downturn but there are clear limits to this approach
[14] https://www-statista-com.ezp-prod1.hul.harvard.edu/statistics/295475/revenue-real-estate-sales-and-brokerage-in-the-us/
Great post! Real Estate is such a challenging space because there are so many factors that are hard to quantify and have a big impact on selling price. I wonder which variables OpenDoor has found to be the most important. I also wonder to what extent OpenDoor has been focusing on “homogenous” housing markets like Las Vegas or Arizona, and avoiding markets like New York and Boston, where there is much more heterogeneity in the housing stock.
Thanks David! Only being active in homogenous housing markets is actually part of the second tenant of focusing in on where the model is most effective – I touched on it briefly above 😉 Interestingly, you guessed exactly the two of the three locations they’re operating in!
Love this analysis. I wonder whether real estate pricing will ever become purely a science though since humans do have irrational tendencies. I’m thinking about a situation where a buyer absolutely has to have the house and ends up paying way higher than the predicted sales price.
Also, if I’m selling my house to OpenDoor, I’d still do some due diligence to make sure OpenDoor isn’t lowballing me, which decreases their value prop of streamlining the research process. But that might just be me.
Thanks Chun! Absolutely agree that there’s a huge emotional component to real estate – particularly family homes. We can certainly see the more scientific approach being very useful for more standard housing stock though. OpenDoor definitely doesn’t try to ‘low ball’ people, but they do price slightly lower than market – it’s up to the seller to decide whether getting a few extra % is worth waiting…
Interesting Post! I wonder what metrics OpenDoor uses to attract its end-consumers given all the other service options in the market. Is it their high sell-through rate? user satisfaction? etc…
Thanks for the comment, Felix. It’s mostly the fact that they can give a seller an offer straight away and close whenever they want to (between 3-60 days). There’s also no financing contingency so the seller gets a LOT more certainty than they would if the market.
Great post Meili! Is the analytic approach from OpenDoor supplemented by people/OpenDoor employees to ensure the accuracy of the pricing output? I am worried about the accuracy of these granular inputs into the model if they are being self-reported by the seller and not an expert that understands the model.
Also, how does OpenDoor turnaround and sell the house? I would imagine that they would need to employee realtors to show the house to prospective buyers since I don’t think we have gotten to the point where people would be willing to make such a large purchase at the click of a button, especially because home buying is so reliant on subjective qualities.
Is this available in New Jersey?
Great questions, Natalie! First, OpenDoor does a physical home inspection to verify the inputs in the model. Second, they allow potential buyers to schedule self-led showings (i.e. no agent takes them to the house). Because the houses are empty, buyers can basically schedule showings to suit their schedule (cf. when you need to make sure the tenant or homeowner is available). However, a lot of negative OpenDoor reviews on Yelp are related to the buying side. Seems like they’re still working kinks out there. Third, haha it is not.
Very interesting post and company! I’m not sure that I accept that housing is more homogeneous in Dallas vs. a place like SF or NYC. Looking at their listings, the houses are very different aesthetically and spread through different areas around the city. There is certainly less variation in pricing (by design). Once the company has refined the model in existing geographies, why couldn’t they expand to a city like SF? The inventory turn would potentially be longer, but given that avg price / sq foot is 5X in SF vs. Dallas, it seems like a huge revenue opportunity.
Thanks, Meredyth! I imagine some of the elements of heterogeneity that they might struggle with in cities like SF and NYC are things like use of the property (many individual properties are rented out to multiple tenants), the nature of the housing (standalone vs condo vs townhouse), the impact of neighbourhood (one block can be priced significantly differently from the one right over – particularly in SF).
Super interesting! Wonder if this has an impact on real estate investors who acquire property for primarily financial gain. We’d probably see prices inflate at a slower pace. Will be interesting to watch what happens to these guys as transaction liquidity that’s critical to their business may be hard to sustain.
Thanks, Amy! Yea, one of the things that isn’t quite clear to me is how this model fits in with a cycle of rising/declining house prices. We’ll have to wait and see!
Interesting post Meili, thanks for sharing! I agree that real estate promises great returns and if they can scale they are model they are likely to be very successful. My concern is about their scalability – you mentioned that they are narrowly focused on single-family hoses priced between $125-500k. This narrow criteria and focus on collecting inputs indicate that they may be relying too much on manual data collection rather than building a robust predictive model. How much do you think can they scale with a labor-intensive data collection process?
Hi Oyku, thanks for your comment! You’re right there is a certain labour-intesiveness to their model but I think that’s something that can’t be completely avoided in the real estate space. At a minimum you’ll still need inspectors to go out and check that the place is structurally sound! Maybe one day, the technology will have developed enough such that, per Andrew’s suggestion, a few photos is all it will take to gather than information.
Thanks Meili! This case reminds me of the CarMax case, where imperfect information in the market caused great transactional costs. While CarMax trained car valuation teams, OpenDoor trained computers to evaluate houses – a much more complex asset.
I’m thinking in the long wrong, say OpenDoor’s model has significant market share, its data input will be feeding on its own output, and create a circular reference, where human price-setters have less influence. Would this still work?
I’m sure it’s not a immediate concern or OpenDoor. But it can be more relevant to other data-driven pricing tools.
Thanks, Hao! Hmm…this circularity is an interesting question. I suppose the mitigating factor is that the market clears where supply equals demand. Hence, even if the model is a bit circular, as long as the market can clear at that price (i.e. the demand from buyers still exist), the business model still works?
Hi Meili,
Your post has had a lot of comments 🙂 – it’s super good.
I may have missed it in your post – but any insight into how much better OpenDoor’s algorithm is vs. Zestimates in OpenDoor’s target markets? Also, how do they generate their proprietary data sets and what are the limitations? I’m kind of skeptical of the sustainability of this competitive advantage because the true nuances of homes seem difficult (but not impossible) to scrape. I could imagine a computer processing publicly available blueprints to determine true square footage, but this power is lost once homes are older and have potentially been renovated. Perhaps their narrow focus is dictated more by the robustness of the data set they can gather in the drill down and not just an issue of heterogeneity. As an example, imagine condos in a single apartment building in NYC that were all constructed in the same fashion but have now been updated many times over by their owners. Could OpenDoor’s proprietary data model ever scale in such a building?